In today's highly competitive eCommerce landscape, delivering personalized experiences is crucial for driving customer engagement, increasing conversions, and fostering brand loyalty. One of the key tools that empower online businesses to achieve this level of personalization is a recommendation engine. In this article, we'll delve into the world of recommendation engines, explore their importance, benefits, potential downsides, and alternative approaches. Let's get started!
What is a Recommendation Engine?
A recommendation engine, also known as a recommendation system or recommender system, is a sophisticated algorithmic tool designed to suggest relevant items or content to users based on their preferences, behavior, and historical data. It leverages machine learning techniques and data analysis to provide personalized recommendations that cater to the unique tastes and interests of individual users.
Supercharge Your eCommerce Campaigns with Replo!
- Ultra-Customizable Landing Pages: Elevate your online presence with Replo's ultra-customizable landing pages tailored for serious eCommerce teams. Craft visually stunning and engaging pages that perfectly reflect your brand's identity and captivate your audience.
- Seamless Integration with Shopify: Say goodbye to manual data transfers. Replo seamlessly integrates with Shopify, pulling data directly from your store. This means you can access your analytics right out of the box, gaining valuable insights to optimize your marketing strategies.
- Pre-built Landing Pages and Templates: Save time and effort with Replo's pre-built landing pages. Choose from a wide range of professionally designed templates that are ready to use, or unleash your creativity by building your own templates from scratch. Achieve stunning results without the hassle.
Unleash the power of Replo and revolutionize your eCommerce campaigns. Maximize conversions, deliver personalized experiences, and unlock your brand's true potential. Get started today and experience the difference.
Why is a Recommendation Engine Important?
As eCommerce teams strive to deliver exceptional user experiences, recommendation engines have emerged as a crucial component of their strategy. Here's why recommendation engines are so important:
Enhanced Personalization
A recommendation engine enables businesses to understand their customers on a deeper level by analyzing their past interactions, purchases, and browsing behavior. By providing personalized recommendations, businesses can offer a tailored experience that resonates with individual users.
Increased Engagement
By suggesting relevant products or content, recommendation engines keep users engaged and interested, leading to longer browsing sessions, increased page views, and reduced bounce rates. This engagement drives conversions and boosts revenue.
Improved Customer Satisfaction
When customers feel understood and catered to, their overall satisfaction increases. By offering personalized recommendations, businesses can make customers feel valued, leading to higher customer retention and brand loyalty.
Cross-Selling and Upselling Opportunities
Recommendation engines identify patterns and correlations between different items, allowing businesses to recommend complementary products or suggest upgrades, thereby increasing average order value and maximizing revenue.
Time and Cost Efficiency
Manually curating personalized recommendations for each user would be a daunting and time-consuming task. Recommendation engines automate this process, saving businesses valuable time and resources while delivering accurate and relevant suggestions.
What are the Benefits of a Recommendation Engine?
Implementing a recommendation engine can yield several benefits for eCommerce businesses. Let's explore some of the key advantages:
Personalized User Experience
Recommendation engines deliver individualized recommendations, ensuring each user receives content or product suggestions tailored to their preferences, increasing the likelihood of engagement and conversions.
Increased Conversions and Sales
By presenting users with relevant recommendations, businesses can guide them towards making a purchase or taking a desired action, leading to higher conversion rates and increased sales.
Improved Customer Retention
Personalized recommendations create a positive user experience, fostering customer loyalty and encouraging repeat visits and purchases.
Better Inventory Management
By analyzing user behavior and purchase patterns, recommendation engines can provide insights into which products are in high demand, allowing businesses to optimize their inventory and stock levels accordingly.
Data-Driven Decision Making
Recommendation engines generate valuable data about user preferences and behavior, which can be leveraged for future marketing campaigns, product development, and business strategies.
Competitive Advantage
Businesses that leverage recommendation engines gain a competitive edge by delivering personalized experiences that stand out from the crowd, attracting and retaining customers in a fiercely competitive market.
The History of Recommendation Engines
Recommendation engines have a fascinating history that has shaped their evolution into a crucial topic in the eCommerce industry. Let's take a brief look at the key milestones:
Early Approaches
The concept of recommendation engines dates back to the late 20th century. The initial approaches relied on simple algorithms like collaborative filtering and content-based filtering to provide basic recommendations.
Netflix Prize
In 2006, the Netflix Prize competition sparked significant advancements in recommendation algorithms. Netflix offered a $1 million prize to improve its movie recommendation system, leading to the development of sophisticated techniques like matrix factorization.
Big Data and Machine Learning
With the advent of big data and advancements in machine learning, recommendation engines became more powerful. The ability to process vast amounts of user data enabled more accurate and personalized recommendations.
Industry Adoption
In the early 2010s, major eCommerce platforms, such as Amazon and Spotify, demonstrated the effectiveness of recommendation engines in driving customer engagement and revenue. This led to widespread adoption across industries.
The Current Environment of Recommendation Engines
In the current eCommerce landscape, recommendation engines play a crucial role in shaping the customer experience and driving business success. Here's an overview of the current environment:
Personalized Experiences
Recommendation engines enable businesses to deliver personalized experiences tailored to individual users' preferences. By suggesting relevant products, services, or content, they enhance customer engagement and satisfaction.
Increased Conversions
Recommendation engines have proven to be effective in increasing conversions and driving sales. By presenting users with personalized recommendations, businesses can inspire impulse purchases, cross-selling, and upselling opportunities.
Enhanced Customer Loyalty
By consistently delivering relevant and personalized recommendations, recommendation engines help build customer loyalty. Customers feel understood and appreciated, leading to repeat purchases and long-term relationships.
Competitive Advantage
In a competitive eCommerce landscape, recommendation engines provide a significant competitive advantage. Businesses that effectively leverage recommendation engines can stand out by offering superior personalized experiences compared to their competitors.
The Future of Recommendation Engines
The future of recommendation engines in the eCommerce industry looks promising, with several exciting developments on the horizon:
Deep Learning and Neural Networks
The application of deep learning and neural networks hold great potential for further improving recommendation engine performance. These advanced techniques can capture intricate patterns and better understand complex user preferences.
Context-Aware Recommendations
Recommendation engines will continue to evolve by incorporating more contextual information, such as user location, social connections, and real-time situational data. This will enable even more precise and relevant recommendations.
Explainable AI
As recommendation engines become more sophisticated, the demand for transparency and explainability increases. Efforts are underway to develop techniques that provide understandable explanations for why specific recommendations are made.
Multi-Modal Recommendations
With the rise of multimedia content, recommendation engines will need to adapt to recommend diverse formats such as images, videos, and audio. This opens up new opportunities for immersive and engaging user experiences.
Ethics and Trust
The future of recommendation engines also entails a focus on ethical considerations, algorithmic fairness, and user trust. Stricter regulations and guidelines may emerge to ensure the responsible use of recommendation systems and protect user privacy.
Are There Any Downsides to Using a Recommendation Engine?
While recommendation engines offer numerous benefits, it's important to acknowledge potential downsides and challenges. Here are a few considerations:
Privacy Concerns
Collecting and analyzing user data raises privacy concerns. Businesses must be transparent about their data collection practices and ensure compliance with relevant privacy regulations to maintain user trust.
Algorithmic Bias
Recommendation algorithms rely on historical data, which can introduce biases if the data itself is
biased. Care must be taken to ensure fair and unbiased recommendations, avoiding reinforcing stereotypes or promoting discriminatory practices.
Overreliance on Algorithms
While algorithms are powerful, they may not always capture the full complexity of human preferences and emotions. It's essential for businesses to strike a balance between algorithmic recommendations and human curation to provide the best user experience.
Technical Implementation and Maintenance
Developing and maintaining a recommendation engine requires technical expertise and ongoing efforts. Businesses need to allocate resources for building, training, and optimizing the recommendation system.
What Are the Different Types of Recommendation Engines?
Recommendation engines can be classified into several types, each employing different techniques and approaches. Here are the main types:
Collaborative Filtering
Collaborative filtering techniques analyze user behavior and preferences to recommend items or content based on the preferences of similar users. It can be further divided into two sub-types:
User-Based Filtering
Recommendations are made based on the behavior and preferences of users who have similar tastes to the target user.
Item-Based Filtering
Recommendations are made based on the similarity between items, suggesting items that are similar to those the user has previously interacted with or shown interest in.
Content-Based Filtering
Content-based filtering focuses on the characteristics and attributes of items or content. It recommends items that have similar features to the ones the user has shown interest in. For example, recommending movies with similar genres to the ones the user has watched before.
Demographic-Based Filtering
Demographic-based filtering recommends items or content based on user demographic information, such as age, gender, location, or occupation. It assumes that users within the same demographic group have similar preferences.
Knowledge-Based Filtering
Knowledge-based filtering recommends items or content based on explicit user preferences and constraints. It takes into account user inputs, such as answering questions or providing explicit ratings, to make recommendations.
Hybrid Recommendation Engines
Hybrid recommendation engines combine multiple techniques to leverage the strengths of different approaches. By blending collaborative filtering, content-based filtering, or other methods, hybrid engines aim to provide more accurate and diverse recommendations.
How Does Google's Recommendation Engine Work?
Google's recommendation engine is powered by advanced machine learning algorithms that analyze vast amounts of user data to deliver personalized recommendations. Here's an overview of how it works:
Data Collection
Google collects user data from various sources, including search history, browsing behavior, location, and demographic information. This data forms the foundation for generating recommendations.
User Profiling
By analyzing the collected data, Google creates detailed user profiles. These profiles include information about users' interests, preferences, search patterns, and interactions with Google services.
Machine Learning Algorithms
Google employs sophisticated machine learning algorithms, such as deep neural networks, to process and analyze user data. These algorithms can identify patterns, similarities, and correlations among users and their interactions.
Collaborative Filtering
Google's recommendation engine utilizes collaborative filtering techniques to identify items or content that are similar to what users have shown interest in. It leverages the collective wisdom of similar users to make personalized recommendations.
Contextual Signals
In addition to user data, Google's recommendation engine considers contextual signals, such as time of day, device type, and location, to further refine the recommendations. This contextual information enhances the relevance and timeliness of the suggestions.
Real-Time Updates
Google's recommendation engine continuously learns and adapts to new user interactions and preferences. It incorporates real-time data to provide up-to-date recommendations and ensure that the suggestions stay relevant over time.
How Can I Build My Own Recommendation Engine?
Building a recommendation engine requires a combination of technical expertise and thoughtful implementation. Here are some key steps to consider:
Define Your Objective
Clearly define the purpose of your recommendation engine and the specific goals you want to achieve. Identify the target audience, the type of items or content you want to recommend, and the desired user experience.
Gather and Prepare Data
Collect relevant data that will drive the recommendations. This includes user data, item data, and any additional contextual information. Ensure the data is clean, organized, and suitable for analysis.
Choose the Right Techniques
Select the appropriate recommendation techniques based on your objectives and available data. Consider whether collaborative filtering, content-based filtering, or hybrid approaches best suit your needs.
Develop and Train the Model
Implement the chosen recommendation algorithms using programming languages and libraries suitable for machine learning, such as Python and TensorFlow. Train the model using your prepared data and fine-tune the algorithm parameters.
Evaluate and Optimize
Assess the performance of your recommendation engine using evaluation metrics such as precision, recall, or accuracy. Continuously optimize the model by experimenting with different algorithms, parameters, and data features.
Implement and Deploy
Integrate the recommendation engine into your existing system or platform, ensuring smooth communication between the engine and the user interface. Monitor the system's performance and gather feedback for further improvements.
What Are the Limitations of Using a Recommendation Engine?
While recommendation engines offer valuable benefits, it's important to be aware of their limitations. Here are some key limitations to consider:
Cold Start Problem
Recommendation engines may struggle to provide accurate recommendations for new users or items with limited historical data. The lack of sufficient data makes it challenging to understand user preferences or find suitable matches.
Overreliance on Popularity
Recommendation engines tend to prioritize popular items or content, potentially overlooking niche or less-known items that may be relevant to individual users. This can result in a lack of diversity in recommendations.
Limited Exploration
Recommendation engines can unintentionally create filter bubbles by reinforcing existing user preferences and failing to introduce new or diverse items. This limits users' exposure to a broader range of options.
Algorithmic Bias
Recommendation engines can inadvertently perpetuate biases present in the underlying data, leading to biased recommendations. Careful monitoring and mitigation strategies are necessary to prevent algorithmic bias.
Privacy Concerns
Recommendation engines rely on user data, raising concerns about privacy and data security. Businesses must handle user data responsibly, respecting privacy regulations and providing transparent data handling practices.
What are the Alternatives to a Recommendation Engine?
Although recommendation engines are widely adopted, alternative approaches can also provide valuable solutions for personalized experiences. Here are a few alternatives to consider:
Rule-Based Recommendations
In this approach, businesses define explicit rules and criteria to make recommendations. While this method lacks the adaptability and personalization of recommendation engines, it can be suitable for simpler scenarios or when specific business rules dictate the recommendations.
Collaborative Filtering
Collaborative filtering recommends items based on the preferences of similar users. It leverages user behavior and preferences to identify patterns and suggest items that users with similar tastes have enjoyed.
Content-Based Filtering
Content-based filtering recommends items based on the characteristics and attributes of the items themselves. It analyzes item descriptions, metadata, or other features to match items with users' preferences.
Hybrid Approaches
Hybrid approaches combine multiple recommendation techniques to leverage the strengths of different methods. By blending collaborative filtering, content-based filtering, or rule-based approaches, businesses can achieve a more comprehensive and accurate recommendation system.
Final Thoughts On Recommendation Engine
recommendation engines have become a vital topic in the eCommerce industry, enabling businesses to deliver personalized experiences, drive conversions, and foster customer loyalty. The history of recommendation engines highlights their evolution from simple algorithms to sophisticated machine-learning techniques. In the current environment, recommendation engines offer a competitive advantage by providing personalized recommendations, increasing customer engagement, and enhancing the overall shopping experience. Looking to the future, advancements in deep learning, context-aware recommendations, and ethical considerations will shape the next generation of recommendation engines.
At Replo, we understand the importance of recommendation engines in the eCommerce landscape. Our platform offers ultra-customizable landing pages designed for serious e-commerce teams. Seamlessly integrated with Shopify, Replo pulls data directly from your Shopify store, empowering you with immediate access to valuable analytics. Take your eCommerce campaigns and editorials to the next level with expertly crafted pre-built landing pages. Start optimizing your recommendations and driving conversions today.
Frequently Asked Questions About Recommendation Engine (FAQ)
Can recommendation engines be applied to different industries?
While often associated with eCommerce, recommendation engines have applications in various industries, including streaming services, social media platforms, news websites, and more. Any business that seeks to deliver personalized experiences can benefit from implementing a recommendation engine.
How can I evaluate the effectiveness of a recommendation engine?
Evaluating a recommendation engine involves measuring metrics such as click-through rates, conversion rates, and user engagement. Additionally, feedback from users through surveys or interviews can provide valuable insights into the effectiveness of the recommendations.
Are recommendation engines solely based on historical data?
While historical data plays a significant role, modern recommendation engines often employ real-time data and contextual information to deliver up-to-date and relevant recommendations.
Can recommendation engines adapt to changing user preferences?
Recommendation engines are designed to adapt and learn from new user interactions. By continuously updating and retraining the algorithms with fresh data, recommendation engines can adapt to evolving user preferences over time.
How does a recommendation engine handle new or trending items?
Recommendation engines typically face challenges when handling new or trending items with limited historical data. To address this, they may use techniques such as content-based filtering or popularity-based recommendations until sufficient data is available to make accurate personalized suggestions.
Can recommendation engines be applied to non-commercial domains, such as content platforms or social networks?
Absolutely! Recommendation engines can be applied to various domains beyond eCommerce. They are commonly used in content platforms, social networks, news websites, music streaming services, and even in personalized learning platforms to suggest relevant content or resources.
How do recommendation engines handle diverse or niche preferences?
Recommendation engines aim to strike a balance between popular and niche items. By leveraging techniques like hybrid recommendations or using diversity-enhancing algorithms, recommendation engines can incorporate diverse preferences into their suggestions, ensuring users are exposed to a broader range of options.
Can recommendation engines be biased or reinforce existing stereotypes?
Recommendation engines can unintentionally perpetuate biases present in the data they are trained on. To mitigate this, it's crucial to regularly audit and address algorithmic bias, ensuring fairness and inclusivity in the recommendations.
How do recommendation engines handle privacy concerns and data security?
Recommendation engines should adhere to strict privacy policies and comply with relevant data protection regulations. User data should be handled securely and transparently, with clear consent mechanisms and options for users to control their data.
Can recommendation engines be integrated with marketing automation tools or email campaigns?
Recommendation engines can be integrated with marketing automation tools or email campaigns to deliver personalized recommendations to users through email marketing. This can help businesses enhance their customer engagement and drive conversions.
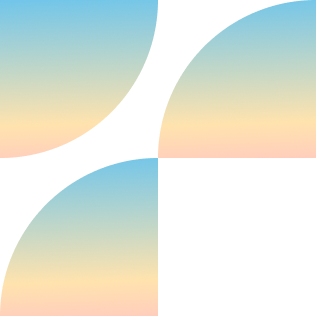
Build, test, and iterate on Shopify without the dev time
Replo has hundreds of templates to help you launch and test new landing pages - without writing a line of code.